The data economy and landscape for 2019
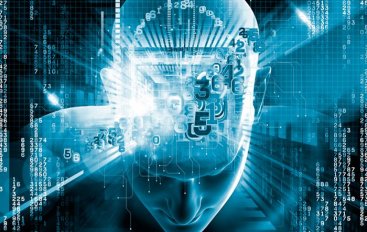
2018 was a year of fundamental change. Underpinning this was the impact of data management and analytics and of course GDPR. Commentary from Cloudera looks back over the year and looks at six key tech areas for 2019…
1. IoT
We have created IoT solutions and edge networks that are far too gullible and trusting.
In 2019, security has to be the number one focus point for organisations to ensure the safety and efficacy of edge devices and networks accordingly. There are too many vulnerabilities and gaps in the security posture for IoT devices -- organisations must take a proactive approach to securing devices. Organizations must use the data, metadata, device logs - treating IoT devices like any other network device to predict and accurately respond to the available signals.
Context is the next major frontier in IoT.
More data islands have been created with IoT, we are now starting to bridge the islands but we don’t speak the same collective language. The ability to acquire data from disparate systems and align it on common ontologies so we can trust and utilize the data. The clockspeed for decision-making is increasing, while information expands exponentially underneath our feet. As AI and machine learning evolve, allowing these capabilities to organize the data, attribute it from a universe of observations, and produce auto-didactic insights, will give us opportunities not yet imagined. Lineage - “what did we know and when did we know it” will be a key capability that allows organizations to use data optimally.
Next year we will see further use cases of IoT in home spaces, smart cities and more industrial use cases in automation or autonomous vehicles. Technology ecosystems are forming so a holistic view of data across the cloud to the edge is important to maximise the benefit of the data used across these ecosystems. Cloudera can do that by making sense of the community and provide the value add and protect the data and the consumer by assuring governance and security.
2. GDPR
The fines associated with non-compliance of the regulation are significant: up to 4% of annual global turnover or $20 million, whichever is greatest. Even if an organisation would not flinch at those kinds of numbers, the impact on their reputation would certainly get them to care about complying. GDPR to a large extend is about showing your customers and employees you are careful with their data, that it is used for the right purpose and that, ultimately, they have control. With that control also comes trust. And any organisation care about that.
Companies made personally accountable for how they treat privacy and personal data
Yes, it is true companies are now personally accountable for GDPR regulated data across the complete data flow, including partners that they need to exchange information with. That also makes it crucial for smaller organisations, suppliers to larger ones, to achieve and maintain their GDPR compliance as it becomes a competitive differentiator.
Big fines ahead for big tech and companies that fail to have adequate security
Data security is but part of GDPR, though an important one: organisations now have the obligation to notify the regulator within 72h of a data breach being discovered. The complete post-breach process including informing the affected individuals is now well defined and following it part of compliance requirements. Under article 25, data protection must be implemented by design and by default; security forms a natural part.
The effects on cloud computing
The effect of cloud computing is such that for organisations, it is important to ensure that the cloud services they use are compliant and that the systems and applications they design do not expose risk.
Do you think GDPR will expand and become a global regulation in 2019?
Expanding GDPR to become a global regulation is a certainly a potential further evolution. Already Cloudera customers and organisations that would not be subject to the regulation are taking it as their starting point for their own personal data privacy and protection guidelines. For it to become a truly global regulation though, it will first need to prove its worth in its current form; once that has progressed well and has proven workable, the chances of it influencing international practice will be much higher.
What organisations subject to GDPR are already realising though is that May 2018 was not the end of the process, the complete opposite. Creating compliance is one thing, but living compliance at scale quite another. What's more, GDPR in its current form may and likely will also evolve further. Organisations that build a solid foundation now, will be able to maintain compliance with less effort as the regulation evolves.
3. Healthcare
80% of all healthcare data is unstructured and for clinicians, doctors, nurses and surgeons, an incredible amount of insight remains hidden away in troves of clinical notes, EHR data, medical images, and omics data to understand patient records better. We are witnessing a revolution in the healthcare industry currently, in which there is now an opportunity to employ a new model of improved, personalized, evidence and data-driven clinical care.
To arrive at quality data, organizations are spending significant levels of effort on data integration, visualization, and deployment activities but organizations are increasingly restrained due to budgetary constraints and having limited data sciences resources.
Healthcare faces many challenges, including developing, deploying, and integrating machine learning and artificial intelligence (AI) into clinical workflow and care delivery. Having the proper infrastructure with the required storage and processing capacity will be expected in order to efficiently design, train, execute, and deploy machine learning and AI solutions. Cloudera is committed to supporting healthcare professionals and institutions to support the next stage of patient care and medical development.
4. Data Warehousing
Data Management goes Cloud?
As more organizations continue to see the economic and ease of use advantages of the cloud we expect to see increased investment in data management in the cloud. Data analytics use cases continue to lead the charge, especially for self-service, transient workloads, and short term workloads. Yet with new technologies that allow us to share data context (security models, metadata, source, and transformation definitions) we will see many organizations grow in use of cloud data management as more than just a compliment to on-premise models, as well as moving to private and hybrid cloud deployments, with greater confidence. New data types will continue to be required to satisfy business analytics, including social media and Internet of Things (IoT), driving the need for inexpensive, flexible storage best served by data management in the cloud. The cloud will also support emerging and new use cases such as exploration (iteratively performing ad-hoc queries into data sets to gain insights through discovering patterns) and machine learning without increasing IT resource demands, fueling further adoption.
5. Machine Learning
We are just at the beginning of the enterprise machine learning transformation. In 2019, we'll see a new step in maturity, as companies advance from PoCs to production capabilities.
Enterprise machine learning (ML) adoption will continue as businesses look to automate pattern detection, prediction and decision making to drive transformational efficiency improvement, competitive differentiation and growth. As early adopters advance from proof-of-concepts to production deployment of multiple use-cases, we’ll continue to see an emergence of technologies and best practices aimed at helping operationalize, scale and ultimately industrialize these capabilities to achieve full transformational value.
Infrastructure and tooling will continue to evolve around efforts to streamline and automate the process of building and deploying ML apps at enterprise scale. In particular, ML workload containerization and Kubernetes orchestration will provide organizations a direct path to efficiently building, deploying and scaling ML apps in public and private clouds. We’ll see continued growth in the automated machine learning (AutoML) tools ecosystem, as vendors capitalize on opportunities to speed-up time-consuming, repeatable chunks of the ML workflow, from data prep and feature engineering to model lifecycle management. Streamlining and scaling ML workflows from research to production will also drive new requirements for DevOps as well as corporate IT, Security and Compliance, as data science teams place increasing demands on infrastructure, continuous integration/continuous deployment (CI/CD) pipelines, cross-team collaboration capabilities, and corporate security and compliance to govern hundreds of ML models, not just one or five, deployed in production.
Beyond technology, we’ll see continuing demand for expert guidance and best practice approaches to scaling organizational strategy, skills and continuous learning in order to achieve the long term goal of embedding ML in every business product, process and service. Visionary adopters will seek to build an investment portfolio of differentiated ML capabilities and optimize their people, skills and technology capabilities to best support it. With our modern, open platform, enterprise data science tools, and expert guidance from Cloudera Fast Forward Labs, Cloudera is focused on accelerating our clients’ journey to industrialized AI.
6. Cloud
As companies understand the value of cloud to their existing infrastructure and applications, choice will become increasingly important. The choice to have a mix of public cloud and on-prem as well as multi-cloud provides companies with the flexibility to choose a solution that best fits their needs. Any vendor that only offers one option and “locks in” a company will find their customers will be at a disadvantage. With this choice of deployment options, the need for a consistent framework that ensures security, governance, and metadata management will become even more important. This will simplify the development and deployment of applications, regardless of where data is stored and applications are run. This framework will also ensure that companies can use a variety of machine learning and analytic capabilities, working in concert with data from different sources into a single coherent picture, without the associated complexity.
These options are part of a larger move to a hybrid cloud model, which will have workloads and data running in private cloud and/or public cloud based on the needs of the company. Bursting, especially with large amounts of data, is time consuming and not an optimal use of hybrid cloud. Instead, specific use cases such as running transient workloads in the public cloud and persistent workloads in private cloud provide a “best of both worlds” deployment. The hybrid model is a challenge for public cloud as well as private cloud only vendors. To prepare, vendors are making acquisitions for this scenario, most recently the acquisition of Red Hat by IBM. Expect more acquisitions and mergers among vendors to broaden their product offerings for hybrid cloud deployments.