Leading the Way into an Embedded World with Machine Learning
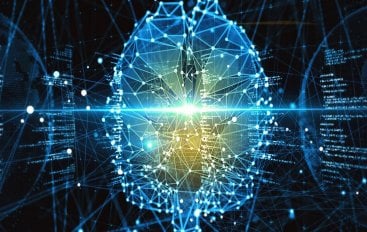
Machine Intelligence (MI) has emerged as the next breakthrough technology. The computing ability to recognize data patterns, accurately identify and classify languages, images, and data types including anomalies such as security breaches are getting stronger every day. Organizations in India are beginning to leverage mature Machine Learning (ML) platforms to improve productivity.
We are seeing an immediate impact in embedded devices that are often located at the edge of the network - think cell towers, robotic arms, and smart city traffic sensors. With MI, embedded smart devices at the edge of the network are rapidly becoming the front line of analysis and decision-making.
This transformation is touching every industry, propelling huge growth and capability for embedded electronics. In fact, it is so important GE spent $1 billion in 2017 alone to analyze data from sensors on their gas turbines, jet engines, oil pipelines and other machines. They aim to triple the sales of their software products by 2020 to roughly $15 billion. Take also the case of retailer, Amazon. They launched their next-generation store, Amazon Go in Seattle last year. The store allows shoppers to take food off the shelves and walk directly out of the store without stopping at a checkout kiosk to pay. The store relies on computer vision to track shoppers after they swipe into the store and associate them with products taken from shelves. When shoppers leave, Amazon debits their accounts for the cost of the items in their bag and emails them a receipt. The store has been well-received by customers in the city and enjoys a 4.5-star rating on Yelp. Given its success, the retailer has launched seven more stores across Chicago and San Francisco in the past year.
Embedded ML applications start from the obvious like unlocking mobile devices with facial recognition for identity authentication. Behind the scenes, security devices such as routers have incorporated smart technology. Now, at the device and network level, real-time analytics analyze traffic and behavior patterns to look for aberrations or signatures that could be security threats.
The impact of ML on the design process of applications can be readily seen with the “Digital Twins” approach. These are digital representations of physical objects, usually specific real-world devices active in the field and represent an exact device configuration at a point in time. By adding sensors to the field device, product designers can gather real-world data and real-time performance. Thus enabled, the device becomes part of the Internet of Things (IoT) and real-world data can feed into ML training sets to improve generative design and facilitate design optimization. It can be used with real-life simulators or serve as a simulated digital twin before a physical design is completed. It can drive not only design improvements but also add whole new capabilities. When running a specific application, designers instantly have visibility into the simulation model in a real-life use case and can see which elements of the design are getting stressed and may need more robust function. Digital twins can spur innovation in any industry, helping companies create a virtual representation of everything it needs to take an idea and create a product.
IoT/Big Data/AI trends will drive the embedded semiconductor market to more than $20B by 2021. These trends are driving demand for networking capacity and compute where it’s needed, at the edge of the network. Real-time processing is often required to handle massive sensor and IOT-generated data. Often, too, the data is simply too large to tolerate the latency of transmitting to the cloud. Edge computing brings high-performance compute closer to data sources. It will take the form of edge analytics, mobile edge compute, smart IoT gateways, and fog compute nodes, all requiring more CPU and GPU compute capabilities with greater networking.
I am more excited than ever about the opportunities ahead of us. This new era of networked connections among people, processes, data and machines will dramatically change how we interact with people and technology. MI will give rise to an entirely new generation of smart products and technologies, embedded everywhere in nearly every manner of device, harnessing data for better productivity. It’s a great opportunity for innovation. Embrace the disruption!