Building Trust & Transparency in AI Matter More Than Ever: Insights from Co-Founder of AI & Beyond
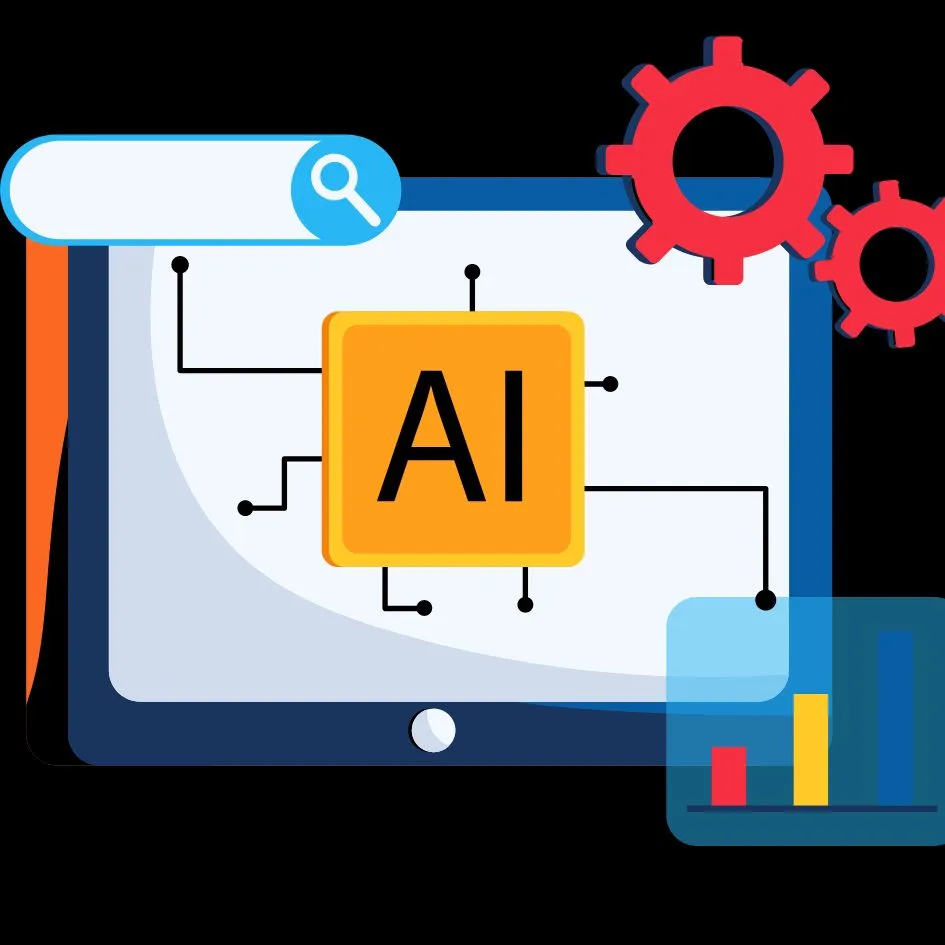
In a world where AI is increasingly embedded in daily life, trust has become the cornerstone of a responsible and sustainable digital future. Over half of consumers view AI as a significant threat to society. Without building trust in AI, both within your organisation and externally, the technology will never reach its full potential or achieve adoption at large. Jaspreet Bindra, Co-Founder of AI & Beyond, shares his thoughts on the crucial elements that define trust in AI, the role of data provenance, and the ethical dilemmas that organisations must navigate to ensure AI systems are not only effective but also transparent, accountable, and empowering for users.
The Vital Role of Data Provenance
According to Jaspreet Bindra, data provenance is the most critical factor in establishing trust in AI systems. Data provenance refers to the ability to trace the origin and transformation of data as it moves through different stages. In today’s digital world, we rely on the accuracy of data to make informed decisions, but as data moves through systems, it often gets transformed, anonymised, or merged with other datasets, making it harder to verify its truthfulness.
Bindra highlights the growing importance of technologies such as blockchain and distributed ledgers to manage data provenance effectively. These systems allow us to trace the exact source of data, ensuring that users can trust the accuracy and integrity of the information. By maintaining a clear record of where data comes from and how it has been altered, organisations can build a strong foundation of trust, particularly in industries such as finance, healthcare, and legal services, where data accuracy is paramount.
Trust and transparency in AI:
In today’s digital landscape, the lack of trust in AI systems is a significant issue. Whether it's due to misinformation, deepfakes, or biassed content, the inability to trust data and AI models has wide-ranging consequences. Jaspreet Bindra emphasises that trust is essential not only in verifying the authenticity of information but also in ensuring that AI systems operate transparently and fairly.
Bindra draws attention to the deepfake phenomenon, where AI-generated fake content can deceive users, eroding trust in the digital platforms we rely on. According to a KPMG survey, 67 per cent of global consumers are concerned about the misuse of AI in spreading misinformation. This demonstrates a growing awareness among users, who are increasingly sceptical of what they encounter online. The challenge for organisations is to create AI systems that are transparent in their decision-making processes and data usage.
Common Concerns at Page:
AI systems are built on vast amounts of data, but as Bindra points out, bias in data and AI models is one of the biggest contributors to distrust. Human beings are inherently biassed, and the data they generate reflects these biases. For example, studies have shown that AI models can reinforce gender or racial biases in applications ranging from hiring algorithms to criminal justice systems. A 2021 study by MIT Sloan revealed that 72 per cent of AI systems exhibit some form of bias, raising serious ethical concerns.
Bindra also discusses the lack of transparency in AI systems. Often, users have no idea where their data is going or how it’s being used. For instance, facial recognition technology is widely deployed in public spaces, but most people are unaware of how their biometric data is stored or analyzed. This lack of transparency, coupled with growing concerns over data privacy, creates an environment of distrust. Data privacy issues, such as the sale of personal data or unauthorised surveillance, are central to the ethical debates surrounding AI. Bindra mentions that incidents of data breaches, hacking, and misuse of personal data only add to this distrust.
Accountability and Integrity in AI:
AI is also a technology and it can't be perfect and free from errors always. Ensuring mistakes are rectified and monitored within can help to win the trust. Accountability is a key factor in building trust in AI, but it’s often obscured by complex legal frameworks and dense terms and conditions that most users never read. Bindra advocates for simpler, more user-friendly consent mechanisms, arguing that the current opt-out approach often misleads users. Instead, users should be given the ability to opt in, making informed decisions about how their data is used. He points to the General Data Protection Regulation (GDPR) or the Indian DPP (Digital Personal Data Protection) Act, as a step in the right direction but stresses that many organisations have found ways around these rules, undermining their effectiveness.
Bindra also raises the issue of accountability in ad tech, where personal data passes through numerous intermediaries. It becomes challenging to pinpoint who is responsible when something goes wrong. This ambiguity makes it difficult to hold organisations accountable for data privacy violations or unethical AI practices. Designating a chief ethics or trust officer can enhance AI processes and build trust. If such a role is absent, ensure someone is tasked with upholding AI integrity.
A Growing Concern:
One of the newer ethical dilemmas emerging around AI is its environmental impact. Training large AI models requires vast amounts of computational power, which in turn consumes significant energy and water. Jaspreet Bindra warns that this is a growing concern, as data centres supporting AI systems contribute to carbon emissions. A study by the University of Massachusetts, Amherst, revealed that training a single large AI model can produce as much CO2 as five cars over their entire lifetime.
This environmental degradation is increasingly at odds with the net-zero commitments many tech companies have made. Both Google and Microsoft, for instance, have recently acknowledged that their AI-driven operations are making it harder for them to meet sustainability goals. As AI continues to expand, addressing its environmental footprint will become an urgent priority.
Balancing Innovation With Ethics:
Beyond environmental concerns, AI also raises broader ethical dilemmas. The issue of plagiarism and copyright in AI models, for example, has led to lawsuits, as models often use content without proper attribution. A prominent case involves The New York Times suing OpenAI for using its articles to train its models without permission. Such cases highlight the complex interplay between innovation and ethical responsibility.
To navigate these dilemmas, Bindra emphasises the need for regulation and proactive design choices. Organisations must address ethical concerns at the design stage, ensuring that AI systems are not only innovative but also aligned with ethical standards. This includes making privacy an opt-in feature by default and designing systems that empower users rather than exploit their data.
Empowering Human:
One of the key points Bindra raises is that AI should augment rather than replace human decision-making. While AI systems are powerful tools, they are not infallible. Ensuring that AI remains a tool for human empowerment rather than a mechanism for control or surveillance is essential. Bindra points out that many countries and organisations are now working to address these concerns through regulations like the AI Act and ethical guidelines that prioritise human agency.
Bindra concludes by identifying capitalism as the most significant barrier to building trust in AI. Large corporations that dominate the AI landscape are primarily driven by profits and shareholder value, which often conflict with user privacy, environmental sustainability, and ethical considerations. The constant drive for growth incentivises companies to monetise data in ways that may not align with broader human values.
To overcome this challenge, Bindra urges leaders to take a values-driven approach to AI development. This means setting a strong example from the top, fostering a culture of trust, and embedding ethical considerations into the fabric of their organizations. Leaders must balance the pressures of profitability with the need to protect user privacy, ensure transparency, and minimise environmental harm.
Bindra believes that trust in AI starts with leadership. Organisations that prioritise transparency, accountability, and ethical responsibility will be the ones that succeed in building trust with their users. By walking the talk, setting clear guardrails, and fostering a culture of trust, leaders can navigate the challenges of AI and ensure that their systems empower users rather than exploiting them. In a world increasingly shaped by AI, these values will be the defining features of a successful and sustainable digital future.